Deep learning detection of nanoparticles and multiple object tracking of their dynamic evolution during in situ ETEM studies
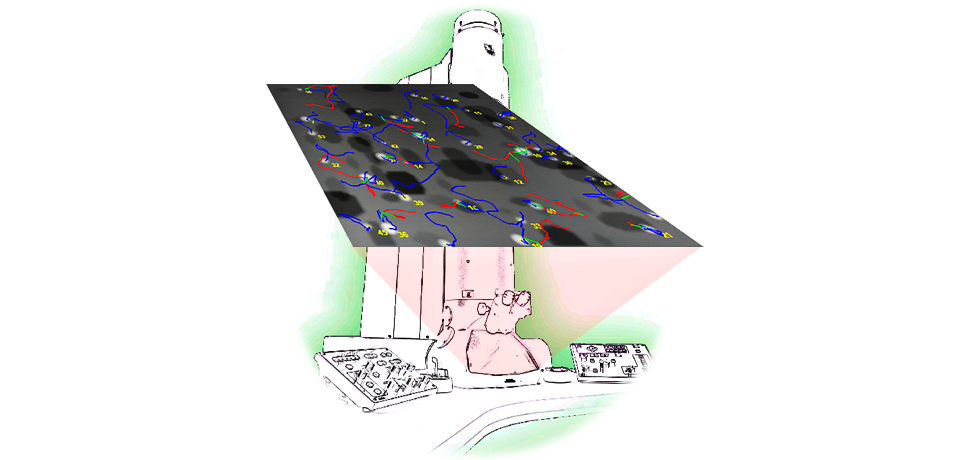
A combined approach by deep learning and computer vision has allowed to quantify automatically the evolution of a population of nanoparticles (NPs) during in situ treatments under gas and in temperature in an Environmental Transmission Electron Microscope.
The video shows a realistic numerical simulation mimicking the motion over time of metallic NPs on a porous oxide support as experimentally observed (2 nm NPs during calcination under oxygen at 250°C). The ground truth is known from the model input data. The treatment by a neuronal network based on U-Net followed by a build-in software NP-Tracker identifies almost perfectly the evolution of NP trajectories (green annotations point out NP coalescence / fusion events).
This work, associating MATEIS, LaHC, CREATIS and IRCELYON from University of Lyon was supported by the IngéLySE CNRS federation and the SLEIGHT EUR.
Khuram Faraz, Thomas Grenier, Christophe Ducottet & Thierry Epicier
Deep learning detection of nanoparticles and multiple object tracking of their dynamic evolution during in situ ETEM studies
Scientific Reports, (2022) 12:2484
https://doi.org/10.1038/s41598-022-06308-2